Welcome to Gabriel-Machado-GM.GITHUB.IO -> My Personal Portfolio
Gabriel Machado - Data Scientist
Objective
Seeking opportunities in Data Science with a focus on Extract, Transform, Load (ETL), Data Analysis, Retrieval Augmented Generation (RAG), Business Intelligence, Machine Learning, and architecting AWS solutions.
Education
-
B.S., Data Science and Artificial Intelligence |
UNIVESP (Universidade Virtual do Estado de São Paulo) (Expected Graduation: 2025) |
Relevant Knowledge & Skills
- Python (Advanced): Object-Oriented Programming (OOP), modularization, file handling, data cleaning, exploratory analysis, supervised (regression, classification) and unsupervised (recommendations, reinforcement learning) machine learning, advanced algorithms.
- Libraries: Scikit-Learn, NumPy, Pandas, Matplotlib, Plotly, Docling, PyTorch, LangChain, MarkItDown.
- Certification: Stanford University & DeepLearning.AI.
- SQL (Advanced): Complex query optimization, function creation, Entity-Relationship Diagrams (ERD), database modeling (relational, key-value, document-oriented, graph).
- R (Advanced): Exploratory data analysis (conditional probability, inference, estimation, hypothesis testing, sampling distributions), data cleaning, processing, and visualization.
- Certification: “The Data Scientist’s Toolbox” - Johns Hopkins University.
- Excel (Advanced): Data organization, filtering, sorting, functions/formulas for cleaning and transformation, statistical/mathematical analysis, charts, pivot tables, macro creation/editing, VBA, scenario analysis, forecasting, interactive dashboards, collaboration.
- Certification: “Excel Skills for Business” - Macquarie University.
- Amazon Web Services (AWS): Practical labs and concepts including EC2, S3, Lambda, RDS, VPC, IAM (Roles, Policies), CloudFront, ELB, CloudWatch, SNS, Route 53. Developed soft skills like Active Listening, Time Management, Effective Communication, and Conflict Management through AWS Re/Start program.
- Certification: AWS Certified Cloud Practitioner.
- Other Big Data Tools: Experience with Google Data Studio / Looker, Zoho Analytics, Tableau, and Power BI.
Work Experience
AI Engineer @ G.A.S. Global Actuarial Solution (2023 - Present)
- Applied AI in Actuarial Expertise. Managed calculation engine parameters (developed in Excel), monitored bugs, implemented improvements, and created auxiliary functions using SQL/Python.
- Innovated by creating a database of legal proceedings (PDFs converted to Markdown) and built Retrieval Augmented Generation (RAG) systems.
- Implemented a complete pipeline for the “Actuarial Expertise” process using Python and libraries like Docling, PyTorch, LangChain, MarkItDown.
Business Intelligence Specialist @ Farol Do Futuro (2020 - 2023)
- Acted as a BI Specialist for 3 years, implementing automated dashboards and reports.
- Analyzed data to optimize processes and strategic decisions.
- Managed databases and KPIs.
- Developed predictive models to identify growth opportunities.
- Collaborated with teams to transform data into actionable insights.
Apprentice Electrical Technician @ Enel Brasil S.A. (2019)
- Performed dimensioning calculations for residential and industrial electrical networks using Excel.
- Created diagrams and projects.
- Studied and applied NBR (Brazilian standards) and NR (regulatory standards).
Languages
- English: C1 Advanced (CEFR) - EF SET Certified (Note: Replace YOUR_CERTIFICATE_ID with the actual ID from the certificate if available, otherwise remove the link)
- Spanish: Intermediate
Volunteer Work
ACM - Alphaville (2016 - 2017)
- Monitor/Collaborator in 12 major events.
- Vice-President of the Leaders’ Body (2017).
- Attended São Paulo Leaders Meeting (2017).
- Attended National Leaders Meeting (2017).
Projects
Data-Driven EEG Band Discovery with Decision Trees
Publication
Developed objective strategy for discovering optimal EEG bands based on signal power spectra using Python. This data-driven approach led to better characterization of the underlying power spectrum by identifying bands that outperformed the more commonly used band boundaries by a factor of two. The proposed method provides a fully automated and flexible approach to capturing key signal components and possibly discovering new indices of brain activity.
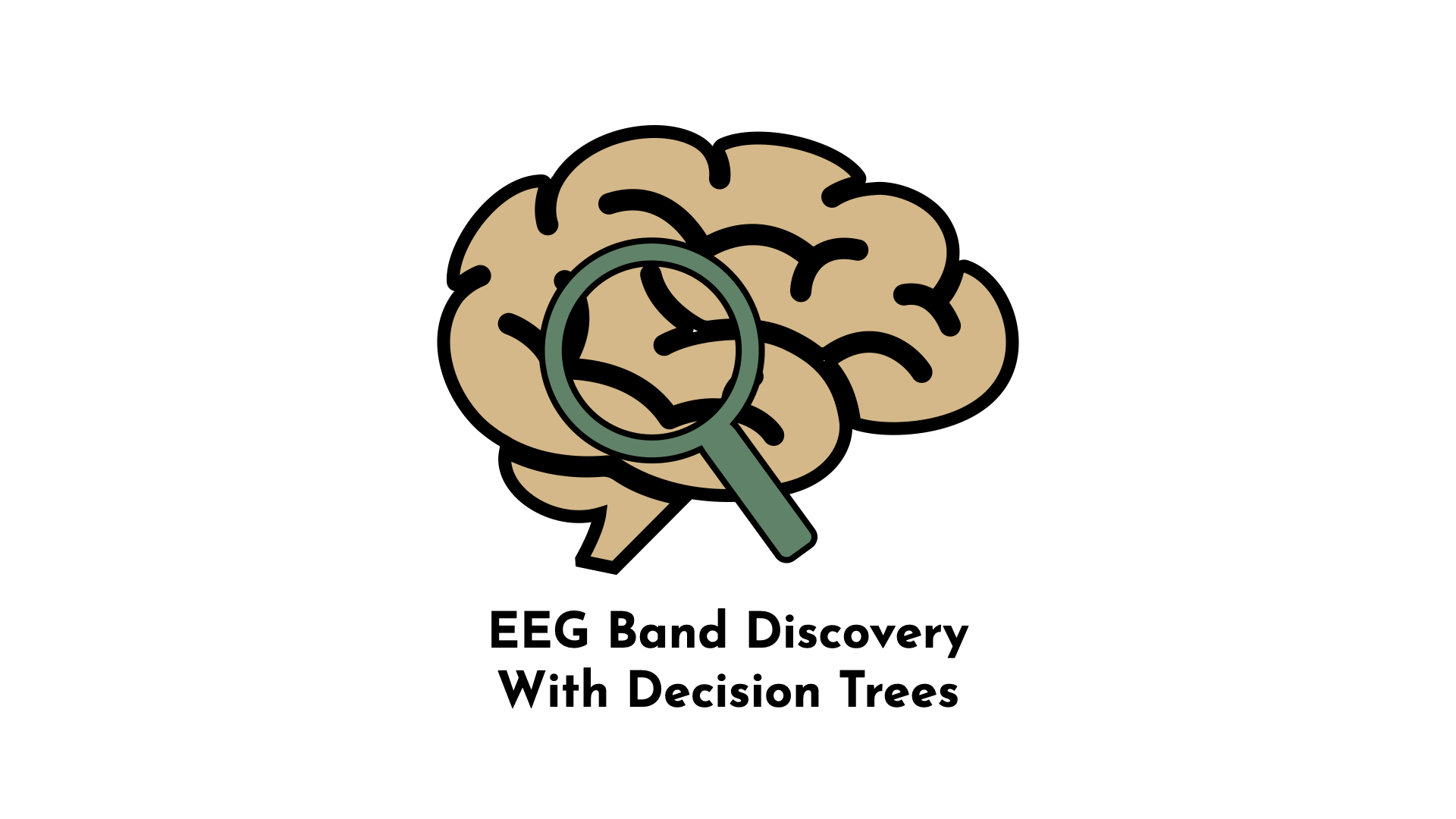
Decoding Physical and Cognitive Impacts of Particulate Matter Concentrations at Ultra-Fine Scales
Publication
Used Matlab to train over 100 machine learning models which estimated particulate matter concentrations based on a suite of over 300 biometric variables. We found biometric variables can be used to accurately estimate particulate matter concentrations at ultra-fine spatial scales with high fidelity (r2 = 0.91) and that smaller particles are better estimated than larger ones. Inferring environmental conditions solely from biometric measurements allows us to disentangle key interactions between the environment and the body.
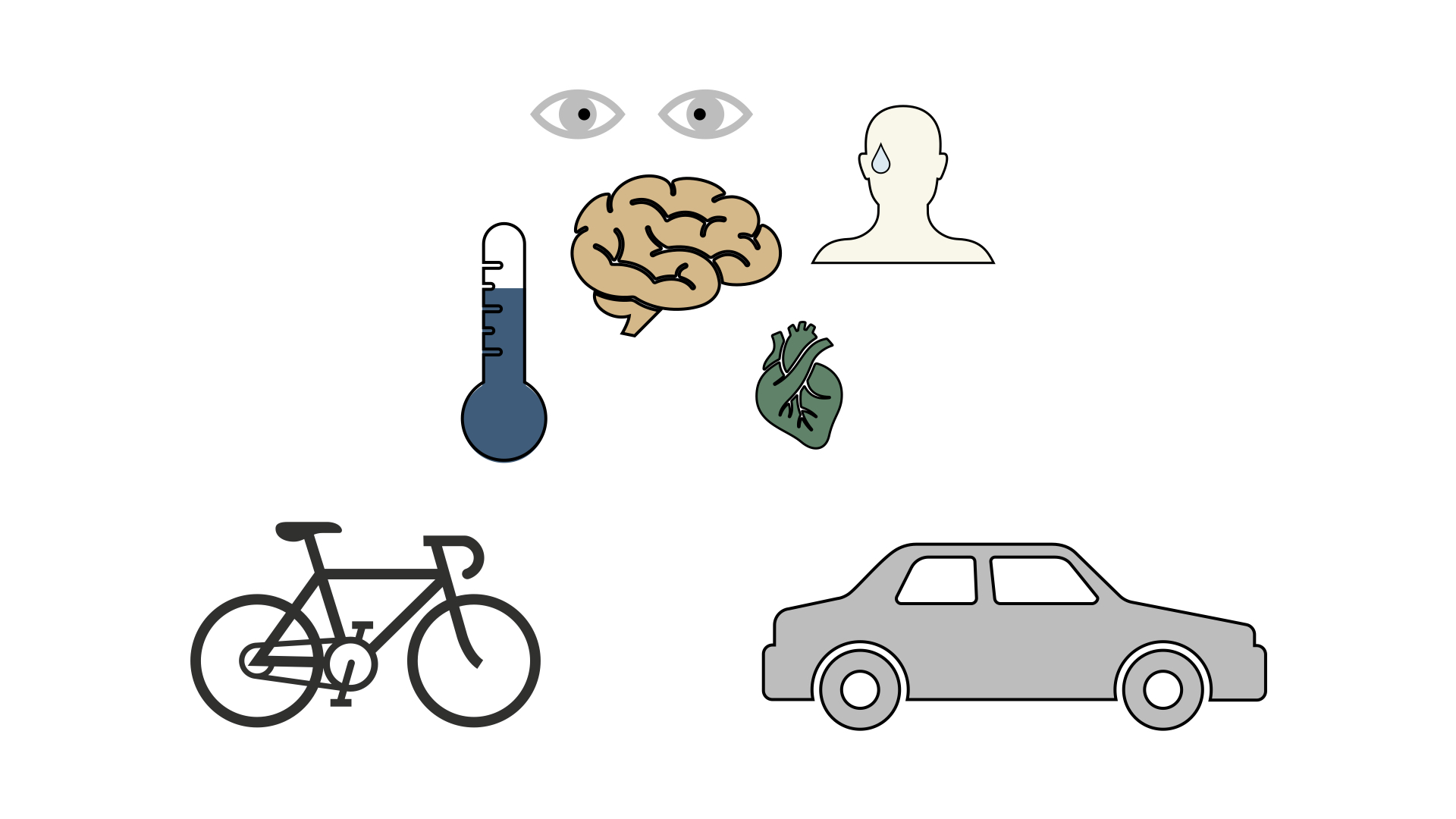
Talks & Lectures
Publications
- Talebi S., Lary D.J., Wijeratne L. OH., and Lary, T. Modeling Autonomic Pupillary Responses from External Stimuli Using Machine Learning (2019). DOI: 10.26717/BJSTR.2019.20.003446
- Wijeratne, L.O.; Kiv, D.R.; Aker, A.R.; Talebi, S.; Lary, D.J. Using Machine Learning for the Calibration of Airborne Particulate Sensors. Sensors 2020, 20, 99.
- Lary, D.J.; Schaefer, D.; Waczak, J.; Aker, A.; Barbosa, A.; Wijeratne, L.O.H.; Talebi, S.; Fernando, B.; Sadler, J.; Lary, T.; Lary, M.D. Autonomous Learning of New Environments with a Robotic Team Employing Hyper-Spectral Remote Sensing, Comprehensive In-Situ Sensing and Machine Learning. Sensors 2021, 21, 2240. https://doi.org/10.3390/s21062240
- Zhang, Y.; Wijeratne, L.O.H.; Talebi, S.; Lary, D.J. Machine Learning for Light Sensor Calibration. Sensors 2021, 21, 6259. https://doi.org/10.3390/s21186259
- Talebi, S.; Waczak, J.; Fernando, B.; Sridhar, A.; Lary, D.J. Data-Driven EEG Band Discovery with Decision Trees. Preprints 2022, 2022030145 (doi: 10.20944/preprints202203.0145.v1).
- Fernando, B.A.; Sridhar, A.; Talebi, S.; Waczak, J.; Lary, D.J. Unsupervised Blink Detection Using Eye Aspect Ratio Values. Preprints 2022, 2022030200 (doi: 10.20944/preprints202203.0200.v1).
- Talebi, S. et al. Decoding Physical and Cognitive Impacts of PM Concentrations at Ultra-fine Scales, 29 March 2022, PREPRINT (Version 1) available at Research Square [https://doi.org/10.21203/rs.3.rs-1499191/v1]
- Lary, D.J. et al. (2022). Machine Learning, Big Data, and Spatial Tools: A Combination to Reveal Complex Facts That Impact Environmental Health. In: Faruque, F.S. (eds) Geospatial Technology for Human Well-Being and Health. Springer, Cham. https://doi.org/10.1007/978-3-030-71377-5_12
- Wijerante, L.O.H. et al. (2022). Advancement in Airborne Particulate Estimation Using Machine Learning. In: Faruque, F.S. (eds) Geospatial Technology for Human Well-Being and Health. Springer, Cham. https://doi.org/10.1007/978-3-030-71377-5_13